
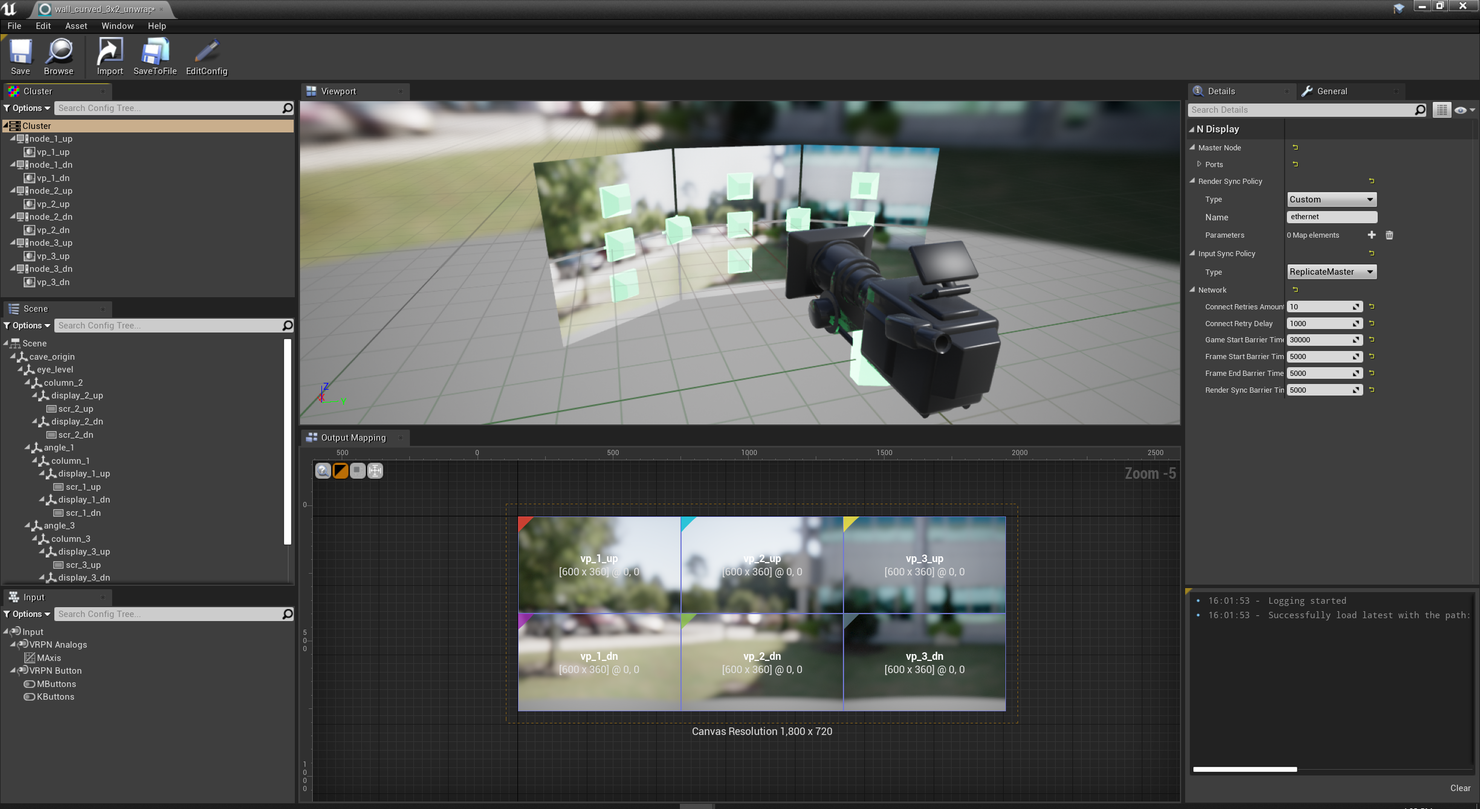
Simulation results with 1-D data and two different imaging applications are presented to demonstrate the efficiency of the proposed schemes.

A practical parameterless version of the GENP-AMP is also developed, which does not need to know the sparsity of the unknown signal and the variance of the GENP. We then focus on GENP-AMP and investigate its parameter selection, state evolution, and noise-sensitivity analysis. We first incorporate the GENP into the LASSO and the approximate message passing (AMP) frameworks, denoted by GENP-LASSO and GENP-AMP respectively. In this paper, we study the compressed sensing reconstruction problem with generalized elastic net prior (GENP), where a sparse signal is sampled via a noisy underdetermined linear observation system, and an additional initial esti-mation of the signal (the GENP) is available during the reconstruction. Index Terms-approximate message passing, compressed sensing, Gaussian mixture model, universal denoising. Our universal CS recovery algorithm compares favorably with existing reconstruction algorithms in terms of both reconstruction quality and runtime, despite not knowing the input statistics of the stationary ergodic signal. In addition to the algorithmic framework, we provide three contributions: (i) numerical results showing that state evolution holds for nonseparable Bayesian sliding-window denoisers (ii) a universal denoiser that does not require the input signal to be bounded and (iii) we modify the GM learning algorithm, and extend it to an i.i.d. sequence by fitting a Gaussian mixture (GM) model. We present a novel algorithm that combines: (i) the approximate message passing (AMP) CS reconstruction framework, which converts the matrix channel recovery problem into scalar channel denoising (ii) a universal denoising scheme based on context quantization, which partitions the stationary ergodic signal denoising into independent and identically distributed (i.i.d.) subsequence denoising and (iii) a density estimation approach that approximates the probability distribution of an i.i.d. Our signals are assumed to be stationary and ergodic, but the input statistics are unknown the goal is to provide reconstruction algorithms that are universal to the input statistics. Abstract-We study compressed sensing (CS) signal reconstruction problems where an input signal is measured via matrix multiplication under additive white Gaussian noise.
